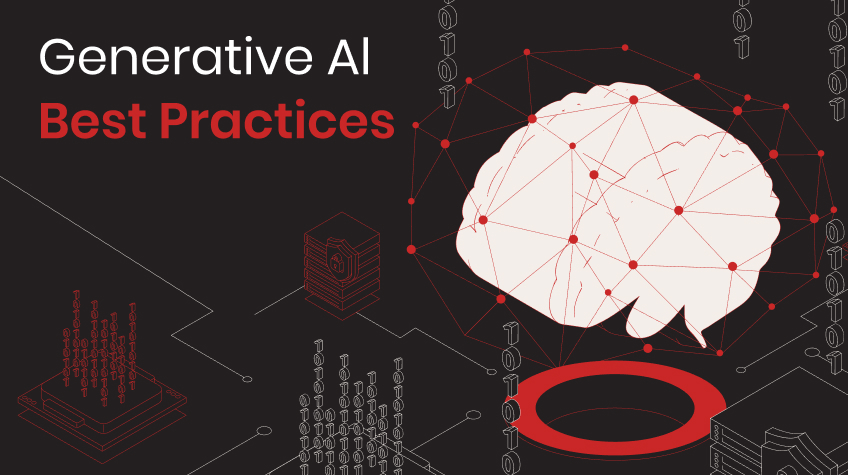
Generative AI is now considered an emerging technology, which provides new solutions in various fields. But for achieving maximum benefits, there should always be the best practices involved. Those practices include having clear goals when developing AI, quality and privacy of data used, controls against bias in outputs from the AI, explaining how the AI is used and so on. Therefore, these practices should be obeyed to enhance the effective use of generative AI while reducing risks.
What is Generative AI?
Generative AI is a form of Artificial Intelligence designed to invent new material, for example, text, graphics, sound, video and even programs from patterns existing in presented data. While ordinary AI is expected to analyze or decide, generative AI generates new content that resembles human creativity.
Supported by state-of-art model architectures including transformers and GANs (Generative Adversarial Networks). It is extensively applied in chatting bots, writing, art and simulation. Its brilliant ability to adapt and create puts it at the forefront of many industries such as entertainment, health care and much more.
How Generative AI Works?
Generative AI, in its most simplified sense, can be explained as using the superstructure of certain advanced machine learning technologies like GANs and transformers to produce new data that imitates the data traits of the original data set. The process flow starts from data training where the learning occurs based on the structure’s relationship and other characteristics within the data. For instance, in text generation, models like GPT use neural networks to predict and consecutively generate textual sequences based on the input provided.
These models use statistical tools to develop output that is realistic and relevant with a view to applying them in writing, art, music and other areas. The main strength of generative AI is the capability to not only recognize but also replicate diverse patterns in data and do so with stunning originality and accuracy.
Best Practice When Using Generative AI
Using generative AI effectively and responsibly requires adherence to the following key best practices:
1. Model Selection for Training Data
In generative AI, it is important to choose the right model for training data. Select the appropriate kind of model for the task, for example, if you work with texts use GPT, for images use GANs and if you work with sounds utilize audio transformers. Make sure that you select a model that is capable of handling the data and task at hand. You may also want to adjust pre-trained models for speed and results.
2. Observe and Assess Results
Observing and evaluating results are essential in generative AI as it ensure outputs are aligned to objectives and still maintain quality. Information that is produced should be constantly checked for reliability, pertinently and for compliance or violation of ethical standards. Coherence, creativity and feedback from the end user should be used as yardsticks to measure the performance of the model and results obtained from this process should be used to make further improvements to the model.
3. Data Privacy
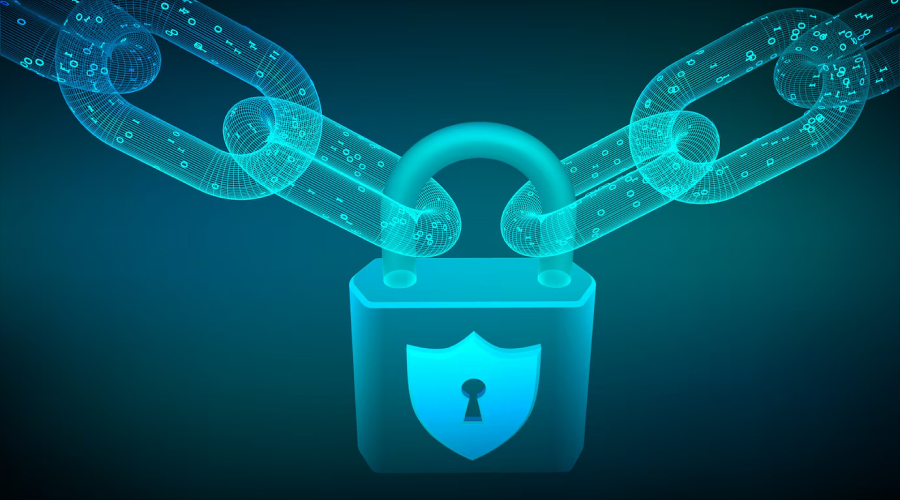
The use of generative AI raised concerns about data privacy. Privacy policies, such as General Data Protection Regulation (GDPR) and CENTRAL CONSUMER PROTECTION AUTHORITY (CCPA), need to be followed by anonymizing the data and asking for the user’s permission.
Through training, we should ensure that other people don’t access the secured resources and during deployment, there should also be stronger security measures to bar people from accessing the secured resources. Be strict on how long data is stored and collected and only focus on the minimal amount of data to avoid exposure to the model’s privacy.
4. Provide AI Transparency
AI transparency means explaining to the end-users how generative AI models function, which data the models synthesize and how the output results are generated. It is best to inform the audience when they are dealing with an AI model and detailing the reasoning the model uses to arrive at a given result is important in creating trust with users. Transparency is also a disclosure of prejudices within the data or model as well as any restrictions or threats of the generated content.
5. Alert For Phishing and Malicious Content
As a measure against phishing attacks as well as objectionable content in generative AI outputs there is a need to put in place blocking of such content. AI models should be trained to avoid the production of nasty and toxic information and the creation of fake and manipulative information.
Employ content moderation tools to bring to light all the recurrent patterns that may need monitoring as well as review all the relations between generated text, images or other media to make sure they meet ethics.
6. Robust Validation Processes
The methods for validation must be very stringent so that the generative AI’s outputs are least erroneous and are legitimate in terms of ethics too. This involves repetitive use of the AI in testing it and checking for errors or biases. Apply mechanized validation strategies like cross-validation and performance indicators and incorporate the approach of peer review together with the assistance of other people to connect the output with the benchmarks of quality and ethics.
7. AI Laws and Regulation
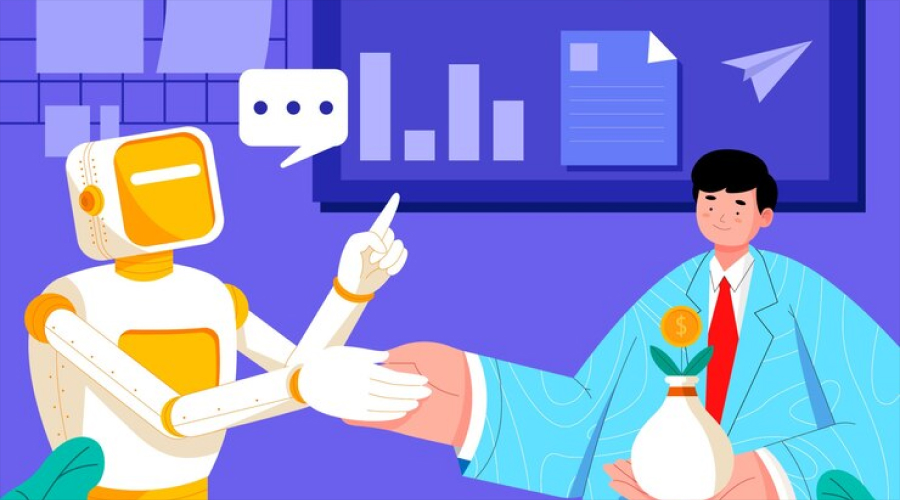
AI laws and regulations are emerging frameworks for the ethical, safe and legal deployment of such technologies. Some of these are related to areas like data protection, explaining algorithms and explaining models, where there is accountability and also where there is bias suppression.
The European Union and the United States are developing AI laws, to promote wholehearted AI adoption but also to regulate them to avoid negative consequences for people. These laws are important to minimize legal issues connected with AI implementation and to establish trust in such systems.
8. Regularly Models Update
Regular model update is needed to ensure relevance, accuracy and efficiency. This entails using new data to train existing models, fine-tuning algorithms and dealing with new trends or threats. Updating is also essential for the model to fit better the real word, correct for biases, perform better and give a better user experience.
Difference Between AI and Generative AI
AI (Artificial Intelligence) is a more general term in computer science, which includes developing systems that can perform tasks typically requiring human intelligence, like problem-solving, decision-making and pattern recognition. AI has many techniques such as machine learning, natural language processing and computer vision.
While Generative AI specializes in classification, prediction and other tasks, generative AI is a kind of AI with a specific objective such as generating new content based on previously existing data in the form of text, images or audio. Its models are the GANs (Generative Adversarial Networks) or transformers, with which it develops its original outputs following learned patterns in large datasets.
➢ Key Differences:
Refer to the table to understand the basic differences between AI and Generative AI
Feature | AI | Generative AI |
---|---|---|
Focus | Generally imitating human intelligence | The focus is on creating new content |
Capabilities | Capable of handling varied tasks including problem-solving, decision-making and learning | More specialized in content generation |
Examples | Self-driving cars, medical diagnosis, fraud detection | Text generation, image synthesis, music composition |
Also Read: Generative AI vs Traditional AI Difference
Conclusion
To sum up, the best methods for using generative AI stress the significance of precise goals, high-quality data, moral considerations and ongoing observation. Organizations may maximize the benefits of generative AI while lowering risks by choosing the best model, maintaining transparency, protecting data privacy and regularly evaluating results.