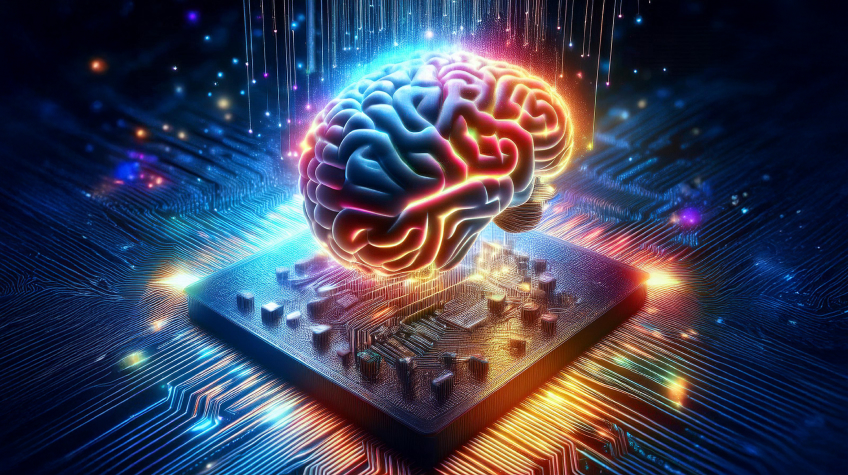
Neuromorphic Computing has recently started gaining acknowledgment, which stimulates the architecture and functioning of the human brain to solve complex computational problems. The technology provides efficient, adaptive, and intelligent solutions for industries, including AI, training robots, machine learning, and autonomous systems, by exploiting brain-like networks. This article will give you insights on what ate the most innovative examples of neuromorphic computing in modern technology.
Neuromorphic Computing Examples
1. TrueNorth
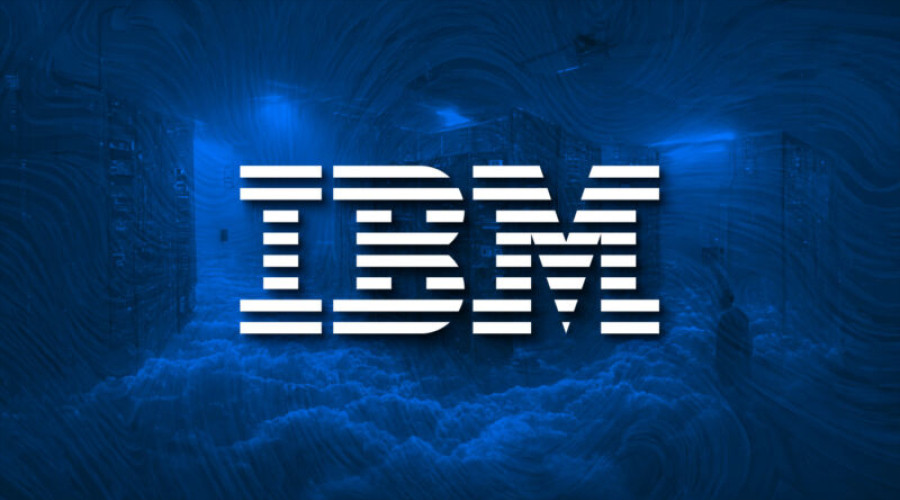
TrueNorth is developed by IBM, it is a neuromorphic chip that emulates a structure in the brain that is very close to an ideal processor AI-like image recognition and machine learning applications, being energy-friendly as opposed to traditional processing units. This chip accommodates 1 million programmable neurons and 256 million synapses, ensuring that massive data can be processed at its core with great energy efficiency.
The architecture of TrueNorth is highly parallel, ensuring that complex tasks are not only carried out but have minimal power consumption. Devices that must run AI tasks need to perform such tasks without delay and with minimal lag, including robotics, drones, and autonomous vehicles. Its development is a considerable step forward toward building the kind of brain-like computational systems for learning and adapting in manners conventional processors cannot.
2. Zeroth
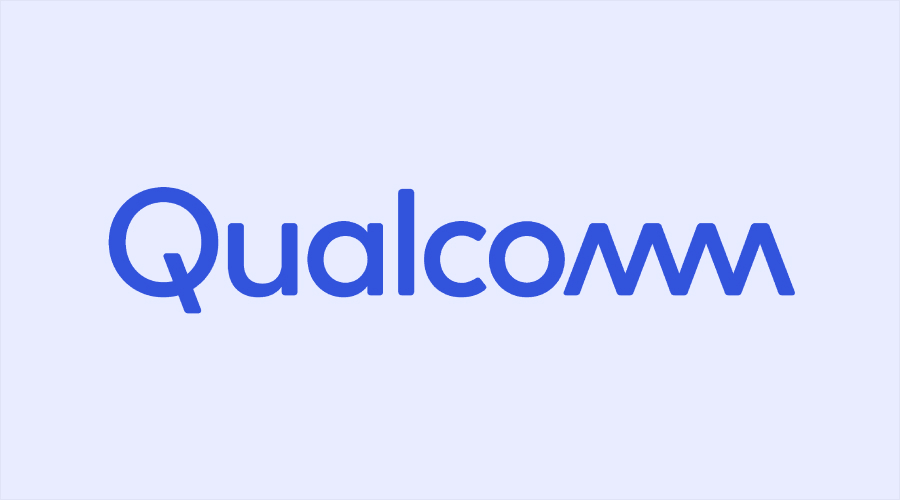
Zeroth is a processor that was developed by Qualcomm and is designed to process sensory data in a human-like manner. With deep learning capabilities, Zeroth excels in real-time decision-making tasks, especially in edge devices like smartphones and autonomous vehicles. The platform enables the development of sophisticated AI applications, such as voice recognition and gesture detection.
Qualcomm’s Zeroth leverages machine-learning algorithms that can process and learn from sensory inputs with minimal latency. This is particularly helpful for mobile devices that need to respond to environmental changes in real-time, providing features such as facial recognition, gesture control, and personalized interaction.
3. NeurONN
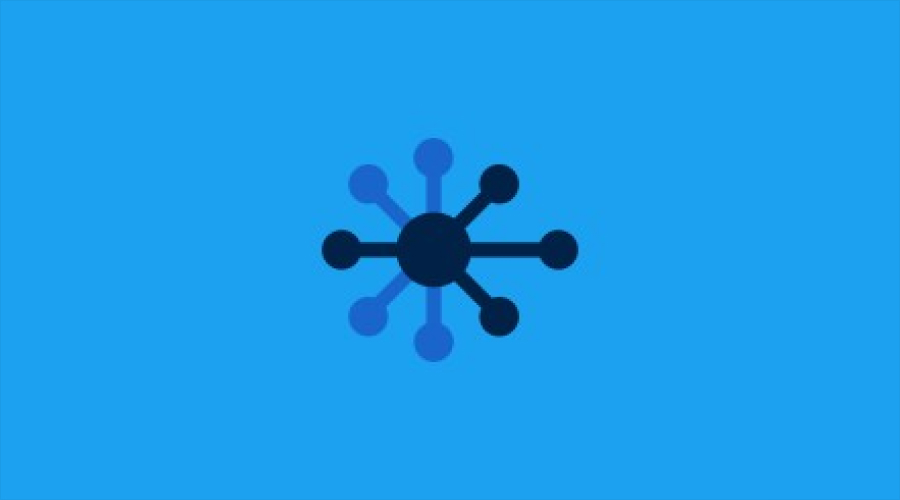
The NeurONN is an EU-funded project of a novel paradigm of neuromorphic computing based on energy-efficient devices and architectures. It follows an ONN approach to encoding in the phase of coupled oscillating neurons to provide inspiration for alternative computation models derived from the functioning of neuronal networks.
The project is led by CNRS (France’s National Centre of Scientific Research) and supported by a consortium of six partners: IBM Research Zurich, Fraunhofer EMFT, CSIC/University of Seville, Silvaco UK, and AI Mergence, France.
NeurONN has also established an Industrial Advisory Board with members from Intel Corporation and Prophesee, thus ensuring strong collaboration between academia and industry to drive advancements in neuromorphic technology.
4. Neuronspike Moore
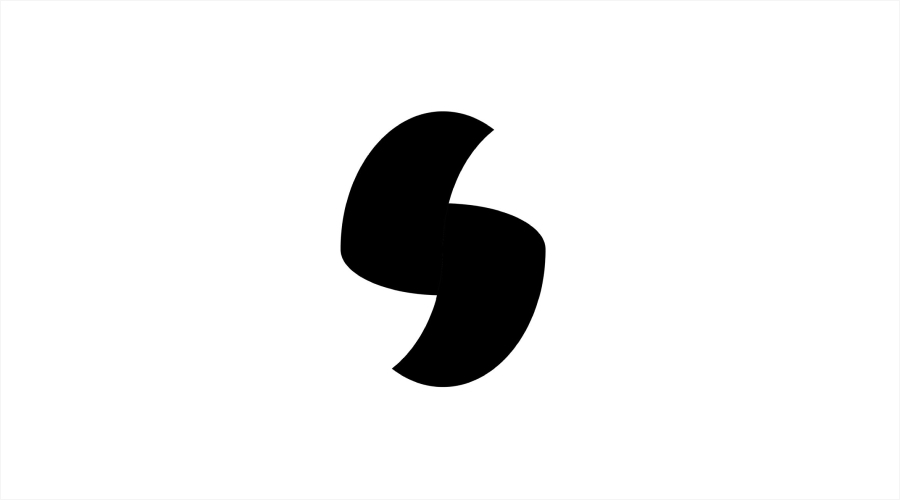
Neuronspike Moore is the world’s fastest LLM chip developed by Neuronspike Technologies. It brings together neuromorphic concepts within a high-performance advanced chip used for machine learning application purposes. It will give room for low-power yet highly performing computation with minimal real-time data processing at the edge.
It has widely been used in the deployment of the edge devices for use since both power efficiency and performance matter. It boosts up to 650 tokens/s in LLM computations with up to 3x more energy efficiency and up to 8x the lower price.
5. Loihi 2
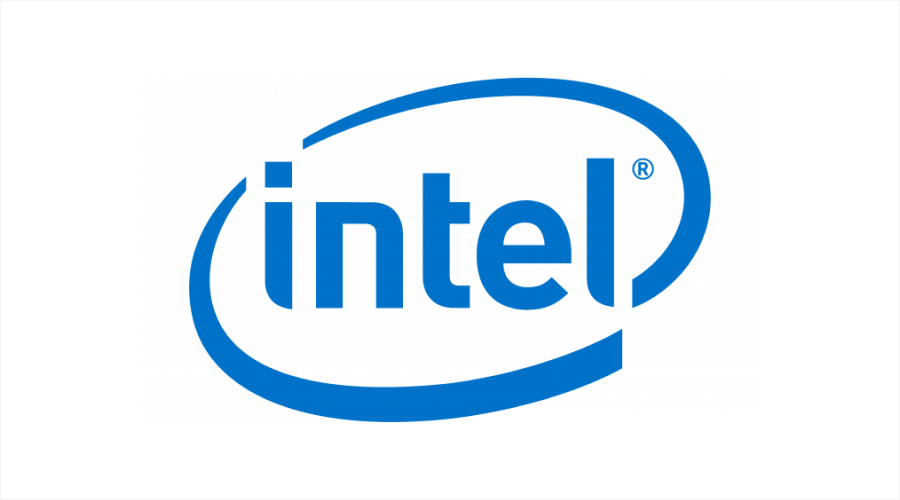
Loihi 2 is a second-generation neuromorphic chip that pushes the boundaries of AI and machine learning. With 1 million neurons per chip, Loihi 2 provides a high level of parallel processing, making it ideal for tasks that require real-time decision-making, such as autonomous systems and sensor data interpretation.
The chip supports unsupervised learning and reinforcement learning, which lets it adapt to dynamic environments and also does not require human input, enabling it to small devices that can perform continuously without recharging regularly, such as field robots, autonomous drones, smart cameras, and several more.
6. NeuroMem Neural Network
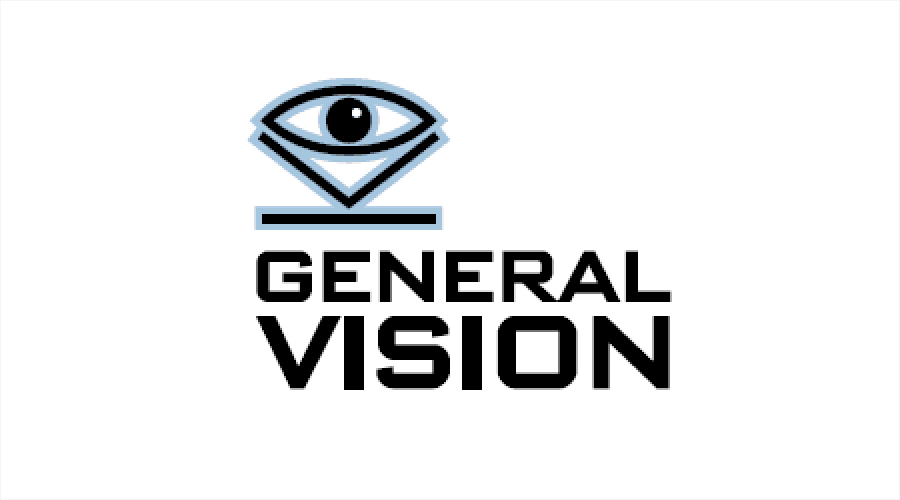
The NM500 chip developed by General Vision Inc. is a fully parallel silicon neural network: it is a chain of identical elements (i.e. neurons) addressed in parallel and which have their own “genetic” material to learn and recall patterns without running a single line of code and without resorting to any supervising unit. In addition, the neurons fully collaborate through a bi-directional and parallel neuron bus which is the key to accuracy, adaptivity, and speed performance. Indeed, each neuron incorporates information from all the other neurons into its learning logic and its response logic.
The neurons can learn and recognize input vectors autonomously and in parallel. If several neurons recognize a pattern (i.e. “fire”), their responses can be retrieved automatically in increasing order of distance (equivalent to a decreasing order of confidence). The information that can be read from a firing neuron includes its distance, category, and neuron identifier. If the response of several or all firing neurons is polled, this data can be consolidated to make a more sophisticated decision weighing the cost of uncertainty or else.
7. The Horizon Europe
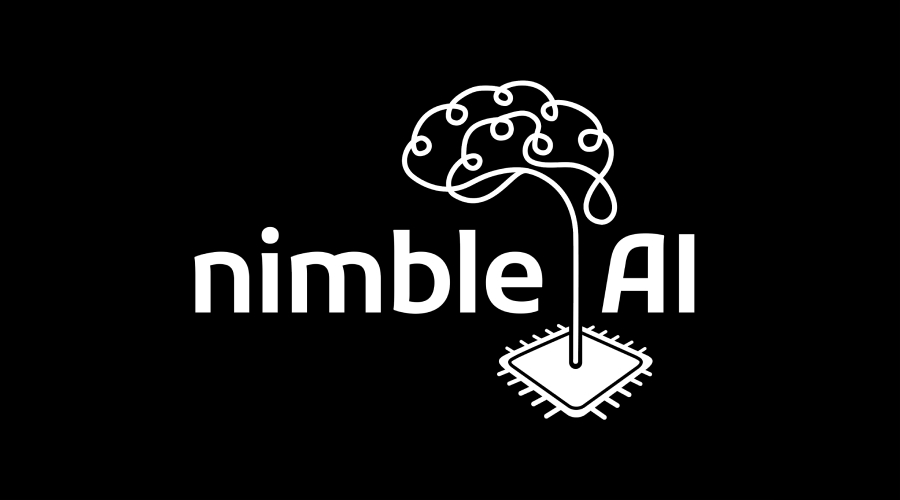
Horizon Europe by NimbleAI neuromorphic hardware and software solutions that utilize European research and innovation. Enables the development of an intelligent system related to specific areas that are healthcare, robotics, and automatic vehicles by setting forth AI models that learn by following sensory data like the brain does in a human.
Horizon Europe seeks the development of Neuromorphic technologies that cross bridges between ML, AI, and computing inspired for the brain. It’s all about sustainability and ethics for AI with this initiative in making a new generation of intelligent systems to be operated autonomously in a different environment, ranging from medical diagnostics to the smart city infrastructure.
8. Akida Neuromorphic System-On-Chip (NSoC)
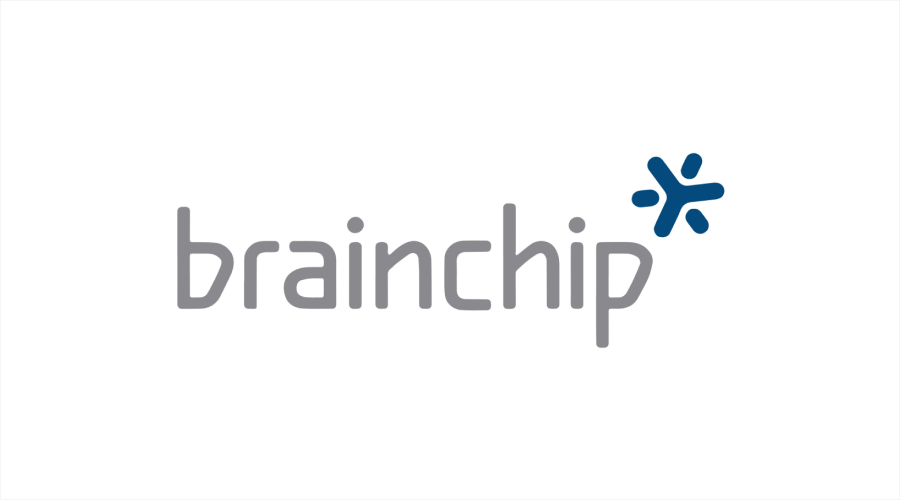
Akida by Brainchip is a neuromorphic chip that integrates AI and machine learning on a single system-on-chip (SoC). This chip uses spiking neural networks (SNNs) to replicate the behavior of the human brain, offering ultra-low latency and high performance for edge AI applications such as smart cameras, drones, and IoT devices.
Akida’s architecture is designed to support both supervised and unsupervised learning, making it flexible for a wide range of use cases. By allowing real-time processing on the edge, Akida reduces dependence on cloud-based computation, which means faster decision-making and better privacy for applications in surveillance, healthcare, and industrial automation.
9. Edge Time Series Processor (TSP1)
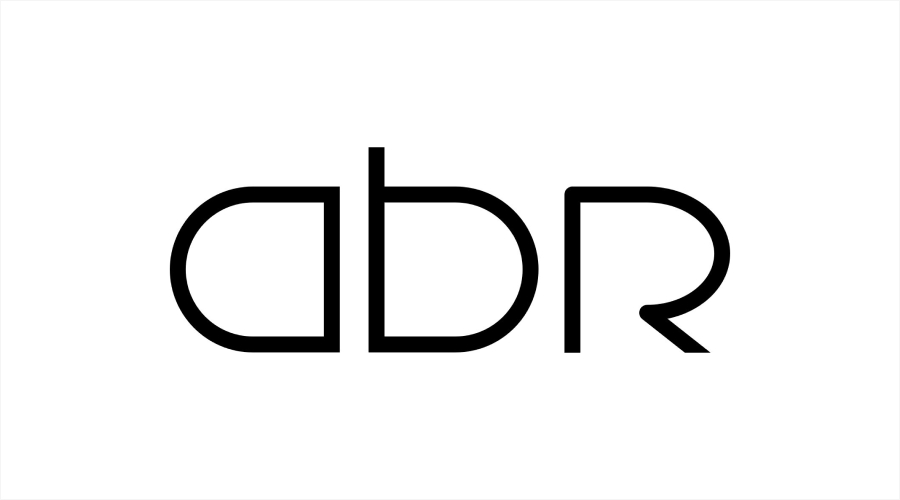
TSP1 processor was developed by ABR for processing time series of data, which has much relevance in predictive maintenance or financial forecasting. This neuromorphic chip is efficient in processing continuous data streams and learning patterns over time, making it a good fit for edge computing applications that require fast, localized decision-making.
The architecture of TSP1 is optimized for detecting anomalies and predicting future events based on past data, which is critical for systems that need to anticipate changes in their environment, such as industrial machinery, smart grids, and financial markets. This means processing time-series data at the edge will enable it to perform real-time predictive analytics without much dependency on a central server.
10. Artificial General Intelligence
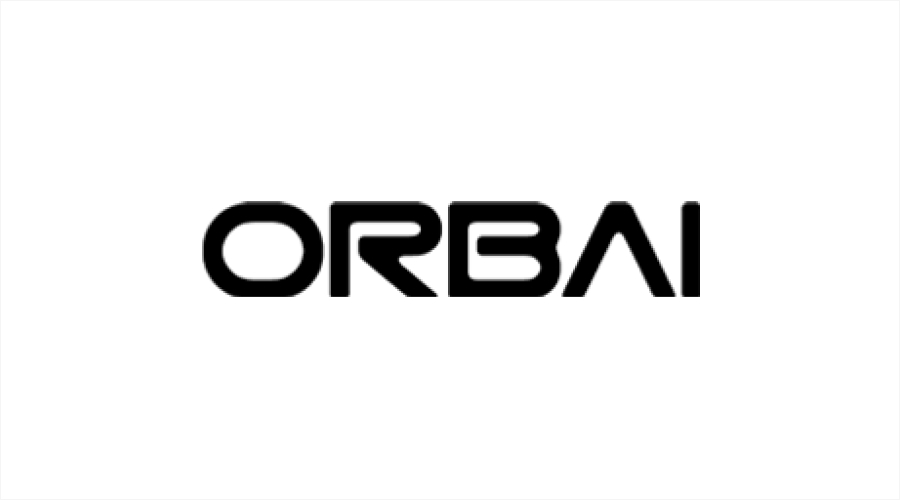
ORBAI is developing artificial general intelligence (AGI) on principles of neuromorphic computing. This replicates the processing architecture of the human brain and can build machines that think and reason about the world in the closest human cognitive manner. Their work has the possibility of revolutionizing industries with genuinely intelligent systems.
Unlike narrow AI, which is specifically designed for a particular task, the neuromorphic computing AGI system can learn from a variety of experiences, adapt to different contexts, and solve novel problems, bringing us closer to machines that can function independently in complex, dynamic environments.
Also Read: Top Edge Computing Examples
11. Neurofin Digital Assets AMC
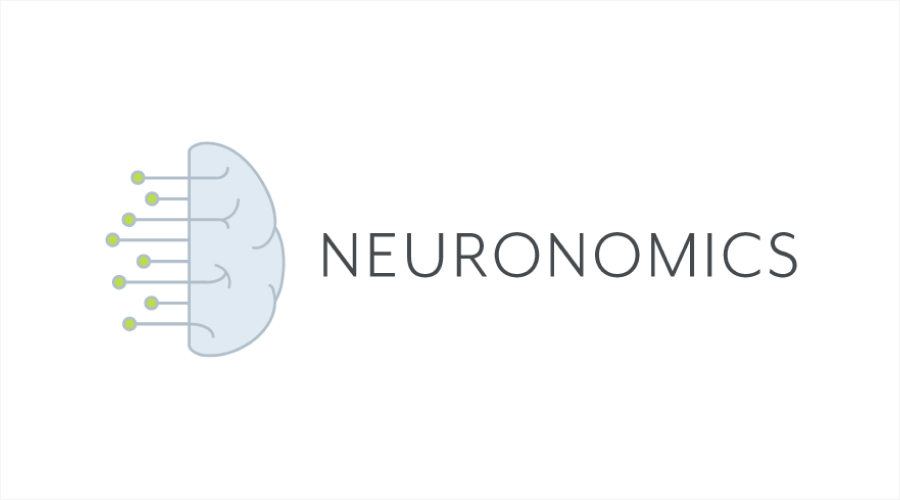
Neurofin by Neuronomics brings neuromorphic computing into the world of digital assets and cryptocurrency. Neurofin provides real-time decision-making systems that adapt rapidly to changing market conditions by applying brain-inspired computing models to financial markets, with innovations in trading algorithms and risk management. It can use historical data to learn market trends and predict extraordinary accuracy movements.
Neurofin’s neuromorphic approach allows it to process vast amounts of market data with much lower power consumption than traditional algorithms, opening the door for more sustainable and scalable solutions in the finance and cryptocurrency sectors.
12. NEUROTECH
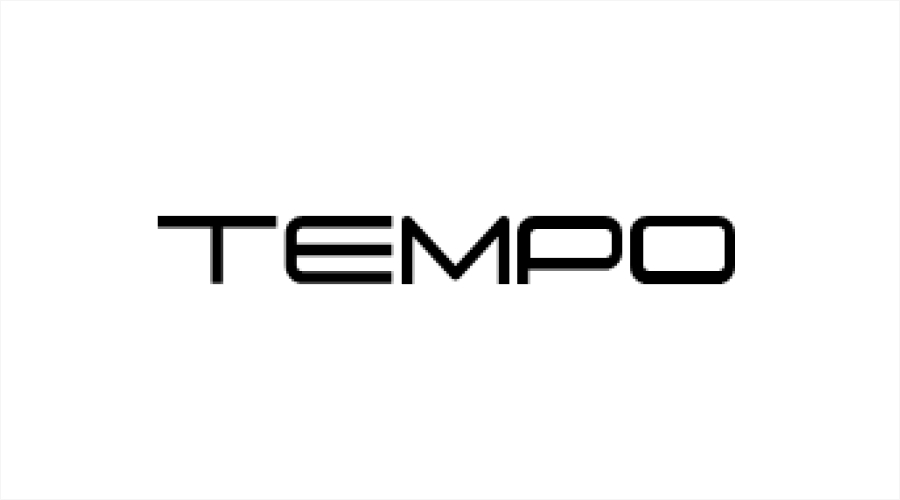
NEUROTECH is a suite of neuromorphic hardware and software solutions by Tempo that aims to revolutionize the AI industry. NEUROTECH is designed to improve cognitive computing with advanced memory and processing capabilities that mimic the human brain, thereby enabling more sophisticated applications in robotics, smart manufacturing, and artificial intelligence.
It combines both analog and digital neuromorphic designs to allow for more flexible and adaptive learning systems. This hybrid model makes it suitable for use in applications that need to operate under a real-time decision-making process with ongoing learning, such as an autonomous robot in a factory, monitoring systems in real-time, and smart AI assistants.
13. differentiable content addressable memory (dCAM)
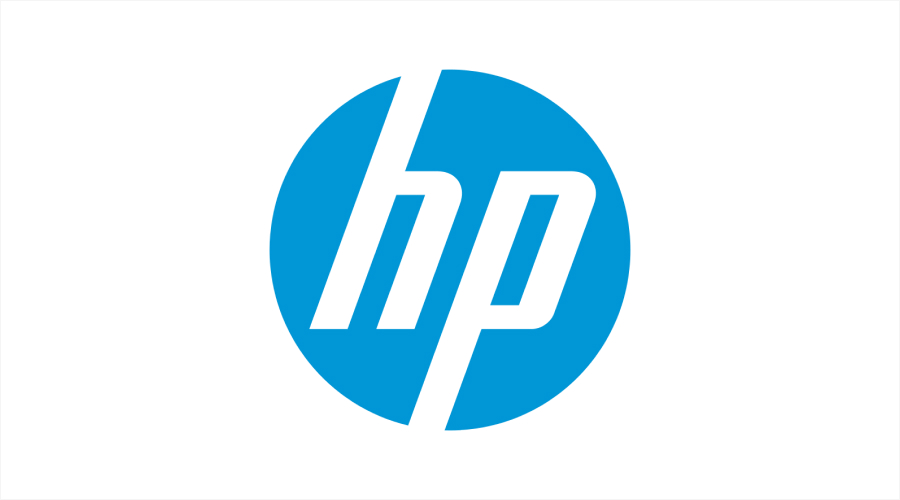
dCAM is a memory technology by HP that integrates principles of neuromorphic processing with traditional computer memory. This technology enables information retrieval with high speed so that it allows more efficient data processing in systems where real-time learning and adaptation are needed, like in AI-driven diagnostics and autonomous systems.
The differentiable property of dCAM enables integration with algorithms used in machine learning, thus allowing the usage of the data stored in the memory during the training process of the neural network. This innovative memory structure considerably enhances the performance of the AI system by removing latency as well as increasing real-time data processing capability for enormous unstructured amounts.
14. Neo NPUs
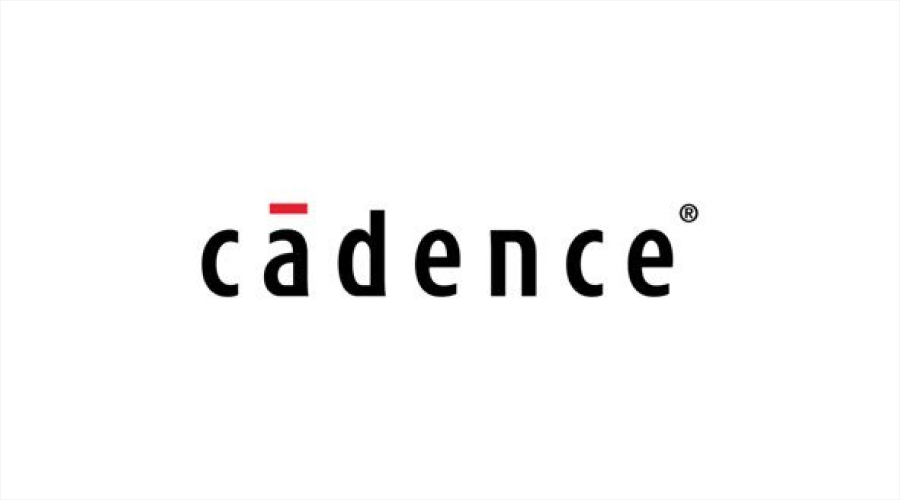
Cadence Design has designed Neo NPUs, which are specialized processors optimized for neuromorphic workloads. These chips are designed to accelerate AI applications especially complex neural networks. It is possible to make real-time AI feasible in mobile and embedded devices by focusing on low-power high-performance processing.
Advanced architectures, these chips are capable of executing traditional deep learning models and even biologically more inspired spiking neural networks; thus, it is appropriate for many applications. Neo NPUs are specifically beneficial for use in areas like speech recognition, image processing, and self-driving because there are strict demands on computation speed as well as low power consumption.
15. Neuroelectronic CMOS Devices
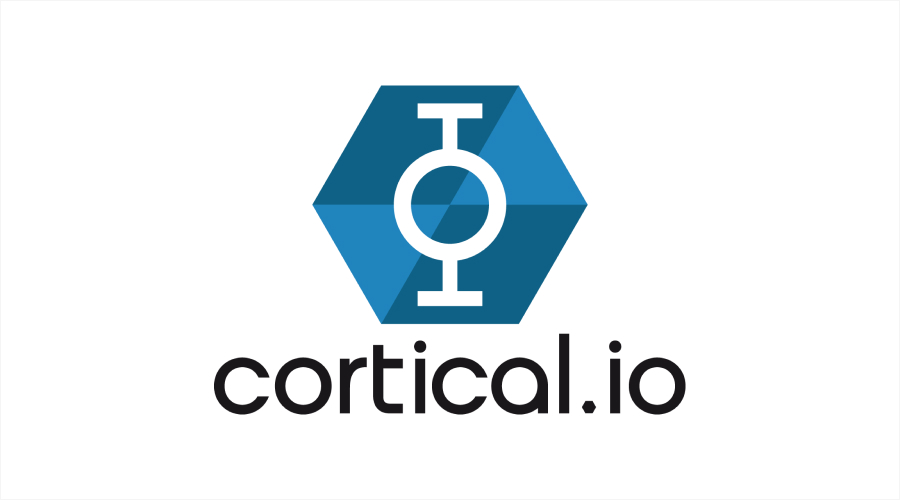
Corticale’s neuroelectronic CMOS devices represent a new wave of neuromorphic computing by utilizing CMOS (complementary metal-oxide-semiconductor) technology for developing neuron-behavior-based circuits. This promises development toward adaptive brain-like systems to learn and react to environmental stimuli, hence offering solutions for AI and biomedical applications.
By combining CMOS technology with neuromorphic principles, Corticale seeks to bring highly efficient and scalable solutions to sectors like healthcare that require real-time monitoring and analysis. Their neuroelectronic devices also create a new form of brain-machine interface and neuroprosthetics for quite more feasible direct communication between electronic systems and the nervous system of humans.
Other Companies in Neuromorphic Computing
Samsung, Accenture, and Knowm are also advancing neuromorphic computing in innovative ways. Samsung is focused on improving memory and processing efficiency for smart devices and IoT applications. Accenture is integrating neuromorphic computing with AI to create adaptive solutions across industries like healthcare and finance. Knowm specializes in developing spiking neural network-based processors for low-power, high-performance real-time AI applications, especially in robotics and predictive analytics.
These companies are driving the next generation of cognitive computing and AI technologies.
Conclusion
Contributed by a large number of companies and research initiatives around the world, neuromorphic computing is evolving very fast, allowing machines to achieve impossible feats such as thinking, learning, and adaptation through advancements in neuromorphic hardware and software.
From systems that emulate brain-cognitive processes to hardware capable of processing complex data efficiently, these innovations are providing the base for smarter, more capable technologies in fields from robotics and autonomous systems to artificial general intelligence. As these technologies advance, neuromorphic computing is sure to dominate and define much of the future of AI and computational science.