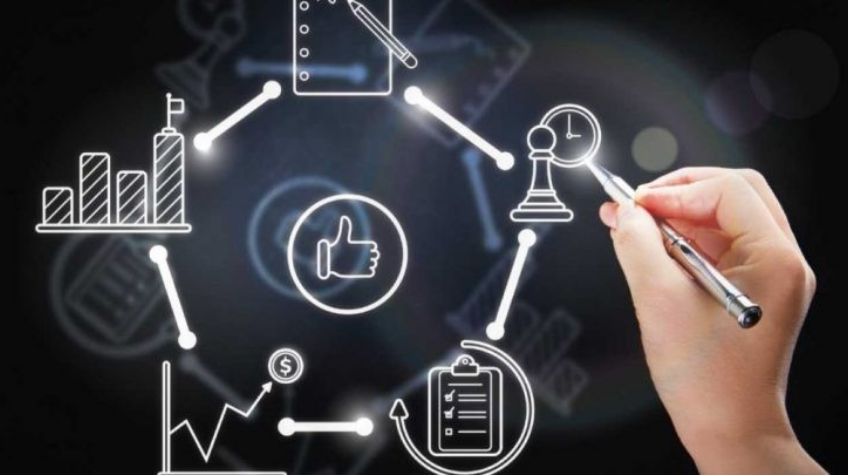
Guide to Statistical Analysis is a powerful tool that helps us make sense of complex data and draw meaningful insights from it. From predicting future trends to identifying patterns and relationships between different variables, statistical analysis enables us to make informed decisions based on empirical evidence.
Whether you’re a researcher, a business analyst, or a data scientist, statistical analysis plays a crucial role in understanding and interpreting the world around us. Using statistical methods and techniques, we can uncover hidden insights that might not be immediately apparent through simple observation.
However, statistical analysis is not a standard process. It requires careful consideration of the data being analyzed, the appropriate statistical models to use, and the limitations of those models. Statistical analysis can provide powerful insights into our world’s underlying patterns and relationships when used correctly. In this blog, we go through the complete guide to statistical analysis.
What is statistical analysis?
Statistical analysis is a methodology used to make sense of data by quantifying and analyzing various features of that data. It involves the application of mathematical models and techniques to explore patterns, trends, and relationships within a dataset. The statistical analysis aims to extract meaningful insights and knowledge from data to inform decision-making, prediction, and hypothesis testing.
There are several critical steps involved in statistical analysis, including:
- Data Collection: The first step in statistical analysis involves collecting relevant data. The data can arrive from different origins, that include research, experimentation, or observational studies.
- Data Cleaning: Once information is accumulated, it must be processed and prepared for analysis. This process removes errors, inconsistencies, and irregularities that distort the analysis outcomes.
- Descriptive Analysis: Descriptive analysis involves using summary information such as average, midpoint, mode, standard deviation, and variance to describe the features of the data. This step helps to identify patterns, trends, and relationships within the dataset.
- Inferential Analysis: Inferential analysis involves driving projections or conceptions about the population established on the model data. This step uses probability theory and statistical models to calculate limits and examine theses.
- Interpretation: The final step in statistical analysis applies to interpreting the outcomes and determinations established on the analysis. This step involves communicating the results clearly and concisely to stakeholders and decision-makers.
Overall, statistical analysis is a powerful tool that enables us to gain insights and knowledge from data. We can extract valuable information to inform decision-making, identify trends and patterns, and support hypothesis testing by applying rigorous statistical methods and techniques.
Types of statistical analysis
Several types of statistical analysis are commonly used in various fields.
Some of the extensively utilized kinds of statistical analysis include:
1. Descriptive Statistics:
- Provides a summary of the data’s main features.
- It incorporates mean, median, mode, standard deviation, variance, and range measures.
2. Inferential Statistics:
- Utilized to make assumptions about a larger population established on a smaller specimen.
- Comprises theory testing, confidence intervals, and reversion calculation.
3. Exploratory Data Analysis:
- Used to recognize patterns and connections in the data.
- Includes scatter plots, histograms, box plots, and other graphical displays.
4. Time Series Analysis:
- Used to analyze data that changes over time.
- Includes trend analysis, seasonality analysis, and forecasting.
5. Bayesian Analysis:
- A probabilistic approach that involves updating beliefs based on new data.
- Includes Bayesian networks, Bayesian regression, and Bayesian inference.
6. Survival Analysis:
- Utilized to examine time-to-event data, like time until failure or recovery.
- Includes Kaplan-Meier analysis, Cox regression, and accelerated failure time models.
7. Multivariate Analysis:
- Used to analyze data with multiple variables.
- Includes primary component analysis, factor analysis, and cluster analysis.
Overall, each type of statistical analysis is helpful for different purposes and can provide valuable insights into the data being analyzed. Choosing the appropriate analysis depends on the research question, the kind of data being scrutinized, and the research objectives.
Importance of statistical analysis
Statistical analysis is essential for several reasons, including:
- Provides insights into complex data: Statistical analysis enables researchers and analysts to make sense of large and complex datasets by identifying patterns, trends, and relationships that might not be immediately apparent through simple observation.
- Informs decision-making: Statistical analysis can provide evidence-based insights in various fields such as business, healthcare, and social sciences. It can assist associations in making better knowledgeable conclusions about resource allocation, marketing strategies, and risk management.
- Tests hypotheses: Statistical analysis enables researchers to test ideas and determine whether the observed data is consistent with the proposed hypothesis. This helps to refine theories and identify areas for further investigation.
- Evaluates the effectiveness of interventions: Statistical analysis is often used to evaluate the effectiveness of interventions, such as drug treatments, educational programs, or public health campaigns. It enables researchers to compare outcomes and decide if the interference effectively impacted them.
- Supports predictive modeling: Statistical analysis can be utilized to develop predictive models to help predict forthcoming tendencies and outputs. These models can be used in finance, economics, and environmental science to make informed predictions and support decision-making.
Statistical analysis is crucial in making sense of complex data, identifying patterns and trends, and informing decision-making across various fields. It enables researchers and analysts to extract valuable insights from data and develop evidence-based solutions to real-world problems.
Benefits of statistical analysis
- Statistical analysis helps to recognize patterns and trends in data that may not be noticeable apart from that.
- It enables decision-making established on data-driven perception instead of instinct or beliefs.
- Statistical analysis can provide a quantitative measure of the reliability of results, giving more confidence in the accuracy of the findings.
- It allows researchers to test hypotheses and evaluate the significance of their results.
- Statistical analysis can help to detect outliers and anomalies in data that might be due to errors or other unusual factors.
- It enables the comparability of different data sets to identify dissimilarities or resemblances.
- Statistical analysis can help to identify relationships between variables, such as cause-and-effect relationships or correlations.
- It can be used to create predictive models, which can be utilized to predict coming movements.
- Statistical analysis helps identify opportunities for improvement or optimization in processes or systems.
- It can be utilized to estimate the significance of interference or treatments in clinical or experimental settings.
Also Read: Impact of SAP Analytics Cloud (SAC) in Your Business Growth
Process of statistical analysis
- Define the research question or hypothesis to be tested through statistical analysis.
- Collect appropriate data, ensuring it is accurate, complete, and illustrates the inhabitants of interest.
- Clean and prepare the data by checking for errors, missing values, outliers, and inconsistencies.
- Choose an appropriate statistical method or model that aligns with the research question and the data analysis type. This may involve selecting from descriptive statistics, inferential statistics, regression analysis, or machine learning algorithms.
- Apply the chosen statistical method or model to the data using software tools such as SPSS, R, or Python.
- Interpret the analysis results, including identifying significant differences or relationships, estimating parameters or effect sizes, and calculating confidence intervals or p-values.
- Validate the findings through sensitivity analyses, robustness checks, or replication studies to ensure they are reliable and robust.
- Draw conclusions and implications from the statistical analysis, including recommendations for action, future research directions, or policy decisions.
- Communicate the results and conclusions of the statistical analysis in a clear, concise, and accessible manner using appropriate graphs, tables, or visualizations.
- Finally, assess the limitations and assumptions of the statistical analysis, including potential sources of bias, confounding factors, or model misspecifications, to ensure that the results are interpreted and used appropriately.
Statistic analysis methods
Here are some statistical analysis methods:
- Descriptive Statistics: Descriptive statistics are utilized to outline and define the main features of a dataset, such as estimates of central tendency (mean, median, mode), variability (range, standard deviation), and distribution (skewness, kurtosis).
- Inferential Statistics: Inferential statistics are utilized to make projections or generalizations about a population established on a data sampling. This contains theory testing, confidence intervals, and regression analysis.
- Hypothesis Testing: Hypothesis testing is utilized to assess the statistical significance of a research hypothesis. It involves specifying a null hypothesis and an alternative hypothesis and testing the likelihood of rejecting the null hypothesis in favor of the alternative hypothesis based on the sample data.
- Regression Analysis: Regression analysis models the association linking one or additional forecaster variables and a response variable. It can be used to make predictions or identify the most critical variables influencing the response variable.
- ANOVA: ANOVA (Analysis of Variance) is a statistical method that resembles means across multiple groups or treatments. It tests whether a significant difference exists in the means of the groups being compared.
- Machine Learning: Machine learning algorithms are used to learn ways and connections in data and to make projections or classifications established on those patterns. Examples of machine learning methods include decision trees, random forests, neural networks, and help vector machines.
- Time Series Analysis: Time series analysis models and forecasts trends, patterns, and seasonality in time-dependent data. It includes methods such as autoregressive integrated moving average (ARIMA) models, exponential smoothing, and spectral analysis.
- Cluster Analysis: Cluster analysis groups similar objects or observations together based on their characteristics or attributes. It can be utilized for buyer segmentation, market research, or data mining.
- Factor Analysis: Factor analysis recognizes fundamental aspects or proportions that describe the variation in a group of variables. It can be used to reduce data complexity and identify the most critical factors that influence a response variable.
Statistics Analysis Software
Here is some statistical analysis software:
- SPSS: SPSS (Statistical Package for the Social Sciences) is a widely used statistical software package for data analysis, management, and visualization. It offers a range of statistical methods for descriptive and inferential statistics, including regression analysis, factor analysis, and cluster analysis.
- R: R is a free, open-source programming language and software environment for analytical computing and illustrations. It offers a wide range of statistical techniques and graphical methods and is highly customizable with a vast array of add-on packages.
- Python: Python is a universal-purpose programming language that has evolved more and more famous for data analysis and scientific computing. It offers a range of statistical packages and libraries, such as NumPy, Pandas, and SciPy, which can be used for data manipulation, visualization, and machine learning.
- SAS: SAS (Statistical Analysis System) is a software suite that provides a comprehensive range of statistical methods and data analysis tools. It is widely used in finance, healthcare, and government industries and offers data management, reporting, and visualization features.
- Stata: Stata is a software package that provides various statistical methods for data analysis, such as regression analysis, survival analysis, and panel data analysis. Exploration in economics, public health, and social sciences utilize it.
- MATLAB: MATLAB is a programming language and computing environment used for numerical computing, data analysis, and visualization. It offers a range of statistical methods and tools, including machine learning algorithms, signal processing, and image analysis.
- Excel: Excel is a broadly utilized spreadsheet interface that offers essential statistical functions and tools for data analysis, such as sorting, filtering, and pivot tables. It can be a valuable tool for small-scale data analysis but may not be as powerful or customizable as dedicated statistical software.
Statistic analysis examples
Here are some examples of statistical analysis:
- A/B Testing: A/B testing is a standard method utilized in digital marketing to analogize two interpretations of a website, email campaign, or advertisement to see which functions sounder. The statistical analysis evaluates the significance of any execution dissimilarities between both versions.
- Medical Trials: Medical trials are experimental studies that evaluate the effectiveness of new drugs, treatments, or medical devices. Statistical analysis is used to compare the outcomes of patients receiving the therapy versus a control group and to assess the statistical significance of any differences.
- Survey Analysis: Survey analysis is used to understand a population’s opinions, attitudes, and behaviors based on a sample of respondents. Statistical analysis is used to summarize the results, estimate margins of error, and test for differences between subgroups.
- Quality Control: Quality control is a process used in manufacturing and production to ensure that products meet specific standards of quality and reliability. Statistical process control methods monitor production processes, detect deviations or defects, and adjust to maintain quality.
- Finance: Finance professionals use statistical analysis to evaluate the risks and returns of investments, estimate the value of financial instruments, and forecast market trends. Methods used include regression analysis, time series analysis, and Monte Carlo simulations.
- Sports: Statistical analysis is used in sports to evaluate player performance, predict outcomes of games, and make strategic decisions. For example, sports analysts may use regression analysis to identify the most critical variables that influence team performance or use machine learning algorithms to predict which players are most likely to be successful in the future.
- Environmental Studies: Environmental studies often involve statistical analysis to identify patterns and relationships in data, estimate ecological parameters, and predict future environmental conditions. Examples include spatial analysis, regression modeling, and multivariate analysis.
- Social Sciences: Statistical analysis is utilized extensively in social sciences, including sociology, psychology, political science, and economics. Methods used include survey analysis, regression modeling, factor analysis, and structural equation modeling.
Related Search: Key Differences Between Quality Assurance And Quality Control
Conclusion
statistical analysis is essential for understanding detailed data and making knowledgeable conclusions established on that data. From medical trials to finance to environmental studies, statistical analysis is used in various fields to identify patterns, relationships, and trends in data and to make predictions.
Statistical analysis software, such as SPSS, R, Python, SAS, Stata, MATLAB, and Excel, makes statistical analysis more accessible and efficient, allowing researchers, analysts, and decision-makers to analyze and interpret data quickly.
In today’s data-driven world, statistical analysis is becoming increasingly important, with the ability to understand and make decisions based on data often being the difference between success and failure. By using statistical analysis to draw insights from data, individuals, and organizations can gain a competitive edge, improve decision-making, and achieve better outcomes. We hope this blog on the complete guide to statistical analysis is useful to the readers.