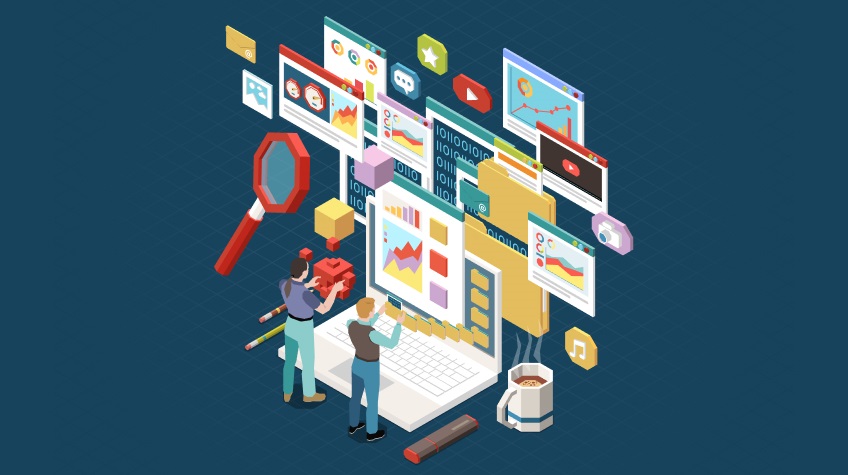
Wondering how data annotation can be beneficial to grow your organization? Since the advancement in artificial intelligence technology, data annotation has become an integral part of most organizations. Everyone is curious to know how a structured and annotated dataset can unleash the capabilities of machine learning algorithms. If you also want to know the advantages and disadvantages of data annotation, then this article is for you. Here, you’ll get to learn a lot about data annotation, including the advantages and disadvantages of this modern technology. So, without any further ado, let’s get started…
Table of Contents
- What Is Data Annotation?
- Features of Data Annotation Tech
- Key Challenges of Data Annotation Tech
- Advantages of Data Annotation Tech
- Disadvantages Of Data Annotation Tech
What Is Data Annotation?
Data annotation is the process of labeling or tagging data so that machine algorithms can easily understand complex datasets. This procedure includes labeling, tagging, and prescribing datasets. Data scientists in this technology label text, images, audio, identify objects in videos, and so much more. It aims to enhance the efficiency of machine learning so that it can generate accurate predictions. For this purpose, it creates a structured dataset to train machine learning algorithms and enhance their speed and accuracy.
Features of Data Annotation Tech
Some common features of data annotation technology are as follows:
➯ Organize Dataset
Data annotation organizes datasets in a structured manner. This is a vital step in the process of training machine learning algorithms. This ensures efficiency and accuracy. The annotated data help machine algorithms in the best possible manner in recognizing, identifying, understanding, and analyzing datasets.
➯ Quality Assurance
Furthermore, data annotation is an indicator of quality assurance. The accuracy of the model for the organization’s function can be measured with the accuracy of the annotated data. In this way, annotated data serves as a standard. Developers and researchers can evaluate the AI model’s efficacy using the annotated data as a benchmark.
➯ Increases AI Model Accuracy
The performance and efficiency of the AI model largely depend on the data annotation. The high quality and precisely annotated data enhances the performance of the AI model. It can learn the desired sequence and patterns in datasets. As a result of this, it offers precise predictions based on the well-structured annotated data.
Let’s now take a look at the key challenges that you may face while data annotation. Take a look…
Key Challenges of Data Annotation Tech
Here are the key challenges of data annotation tech that you should know. Take a look…
➯ Annotation Quality
To avail of the benefits of machine learning algorithms, this is essential that annotated datasets are of high quality. Any degradation in the quality directly can adversely impact the performance of ML algorithms.
➯ Good Annotators
The second challenge is to find highly qualified data annotators for this purpose. Annotating data is not an easy task, especially if there are no good annotators. Any misleading dataset can lead to incorrect outputs. However, there are many annotators, but finding a skilled and reliable annotator is always a challenging task.
➯ Data Privacy and Security
There always remains a risk of digital crooks intervening or hampering in the procedure of data annotation. Any infected malware virus can infect the whole system. Data stealing of sensitive information can result into severe consequences.
➯ Subjective Labeling
The issue of subjective labeling also persists in data annotation. There always remains a chance to encode invalid or unreliable information into the machine algorithms. This subjective labeling makes the output unreliable and biased for users in the end.
Let’s now take a look at some of the most promising advantages of data annotation tech. Here we go…
Advantages of Data Annotation Tech
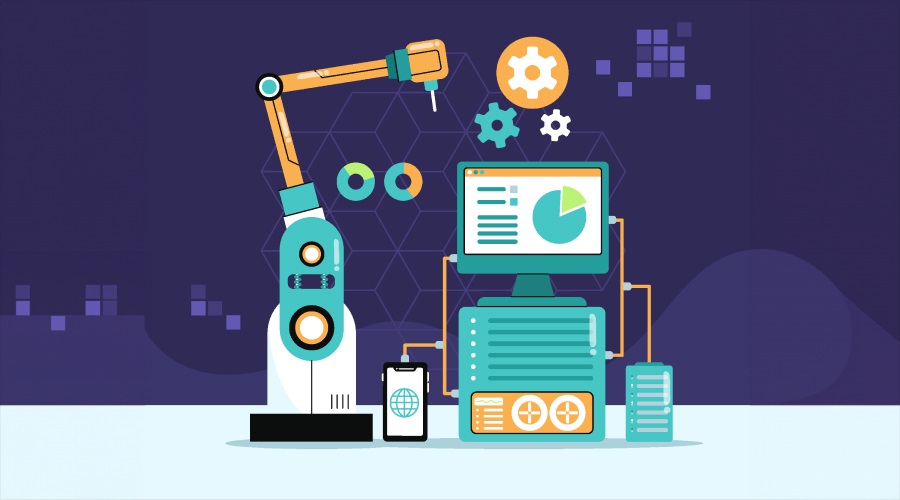
Data annotation is a very advantageous technology, especially in the era as a wide number of companies now depend on AI. Here are some of the advantages of data annotation. Take a look…
➯ Improved Accuracy
The accuracy and reliability of datasets of different machine algorithms depend on the huge datasets. In such a situation, data annotation comes in handy. Data annotation organizes and tags datasets into different components. It ensures that the output that machine algorithms give is accurate and reliable.
➯ Enhanced User Experience
User experience MATTERS! Everything that an organization does is for improved user experience. Data annotation comes in for rescue when it comes to providing users with a mesmerizing experience. This technology helps organizations in developing automated applications.
Chatbots or virtual assistants function based on the annotated information. Bots and virtual assistants help organizations in improving user experience. Virtual assistants can help users with prompt information, solve their queries instantly, and are available 24×7. Ever since the introduction of chatbots and virtual assistants, users have been able to have personalized experiences.
➯ Save Time and Energy
Good data annotation reduces the need for correcting errors or flaws. This saves a lot of time and energy for organizations. Organizations are able to reduce the time that it takes to develop AI models that are compatible with their brand. Consequently, the organization can use this saved time and energy to have a competitive edge.
However, like they say every coin has two sides, data annotation technology also presents some disadvantages, which we are going to discuss in the upcoming section, so keep reading.
Disadvantages Of Data Annotation Tech
Go over the following pointers to know the disadvantages of the data annotation tech:
➯ Inaccuracy
No matter whether you use a data annotation tool or manual annotation, data annotation often becomes subject to error and inaccuracy. Inaccurate annotations can lead to the problem of a biased dataset and inaccurate predictions.
➯ Costly
Data annotation is an expensive technology. One of the reasons of data annotation being expensive is the requirement of highly qualified professionals. Yes, only highly qualified data scientists or engineers are able to perform data labeling or tagging to train machine algorithms.
Due to the relatively recent emergence of ML technology, there is a shortage of professionals available to handle data annotation tasks. The higher charges for data annotation jobs by data scientists contribute to the overall cost of this technology, making it quite expensive. Moreover, data annotation requires high-quality data annotation tools to effortlessly label or tag datasets. These tools come with a price, which again adds to the overall cost for the organization.
The Bottom Line
To sum up, data annotation has revolutionized Artificial Intelligence. The reason behind the escalating AI’s market size is the labeling or tagging of the dataset. Data annotation tech is fundamental to the development and success of AI and ML. It offers significant advantages such as improved accuracy, enhanced user experience, and the ability to automate complex tasks. This, in turn, fuels progress and effectiveness in a wide range of industries, spanning from healthcare to autonomous vehicles.
Nevertheless, data annotation does come with its fair share of challenges. These challenges include the requirement of significant time and resources, the possibility of biases in annotated data, and the dependence on human expertise for quality control. It is essential to carefully consider both the advantages and disadvantages in order to maximize the effectiveness of data annotation tech.
By overcoming obstacles and dedicating resources to cutting-edge technology and expert annotators, you can unleash the complete capabilities of data annotation. This will help develop AI apps that are more precise, dependable, and uphold ethical standards. Also, it is crucial to choose highly skilled and qualified professionals for the task. Doing so will save you a lot of energy, time, and of course, money.
Let’s now take a look at some of the frequently asked questions related to data annotation technology. Here we go…
FAQs
Q1. Is data annotation a unique skill?
The quickest answer to this is YES! Yes, it is crucial for Data annotators to be highly skilled and experienced. They should be able to pay attention to even the minute details of the dataset. When it comes to machine learning, paying attention to the minutiae can help ensure that the datasets are correct and consistent.
Q2. Can data annotation be done with automated tools?
In a way, YES, data annotation can be done using automated tools. In fact, there are several automated tools available out there to use. Some of the tools are, in fact, very efficient in labeling or tagging keywords on datasets. Automated data annotation tools utilize machine learning algorithms to label data quickly and efficiently, which ultimately reduces the time and effort required compared to manual annotation.
In addition, these tools can handle large volumes of data. It ensures faster development. Furthermore, automation can help standardize the annotation process, thereby minimizing human error and increasing consistency. However, while automated tools are highly efficient, there is a chance of incorrect labeling. They cannot provide accurate results the way human annotators can provide, especially in complex or ambiguous cases. Therefore, it is suggested that automated tools and human oversight be combined for the best outcomes.
Q3. Can anyone do data annotation?
Data annotation requires specialized skills. Not everyone can annotate datasets, especially complex ones. Only expert hands can annotate complex datasets with their specialized skills in this domain. The quality and effectiveness of the annotations depend significantly on the annotator’s knowledge and understanding of the task. An annotator must be trained and experienced in the field to justify the role.
Data annotation requires attention to detail, consistency, and detailed understanding of the subject matter to accurately label data. Without proper training and knowledge, annotators won’t be able to perform the task properly. There will always be a risk of errors and biases in the process. This can lead to subpar quality of the dataset, which can adversely impact the performance of machine learning models. Therefore, it is crucial to get proper training in the field prior to taking a data annotation role.