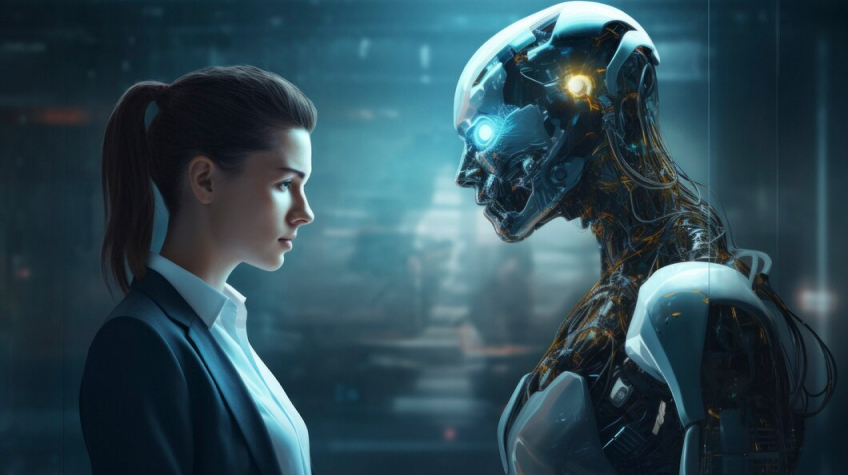
With the ever evolving landscape of generative AI, organizations are transforming the way they do business and meet their customers’ satisfaction. However, in the absence of data science or deep AI expertise, integrating AI powered models into existing workflows is not as easy as it seems.
As a result, it has captured the interest of companies in various sectors. Therefore, it becomes vital to understand how generative AI should be used intelligently and strategically in the modern business landscape.
Read this article to learn how to enhance the capabilities of generative AI to improve business efficiency and enhance personalized customers’ experiences.
Let’s get started…
What is Generative AI?
Generative AI or generative artificial intelligence, refers to deep learning models that generate computer code, human like voices, texts, images, videos, synthetic data sets and complex virtual environments. Significantly, it blurs the line between what is clear and what machine algorithms generate.
Generative AI uses trained data to create artificial things like modern AI forms. It emphasizes using databases to form more data based on their learned patterns and structures. This way, generative AI provides more opportunities for organizations allowing them to add more value to their brand.
However, generative AI can introduce many risks with its usage like copyright issues, biased information, job threats, etc. Hence, organizations are looking for its vigilant usage these days.
Let’s now know why Generative AI is crucial for businesses in the age. Here we go…
Importance of Generative AI for Businesses
Without a doubt, generative AI adds more value to businesses by providing more opportunities to grow and satisfy their customers’ experiences. Check out the following pointers to learn why it is crucial for enterprises:
- It automates repetitive tasks allowing team members to focus on what matters the most.
- It creates personalized products or services for customers, ensuring their high satisfaction.
- It accelerates innovation and productivity by suggesting the blueprint allowing enterprises to achieve a competitive edge.
Understanding Generative AI Management
1. AI Model Lifecycle
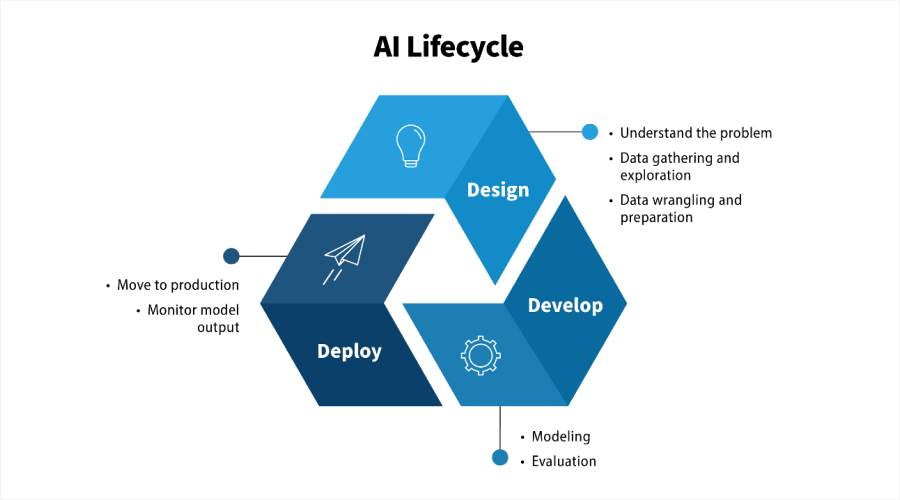
Here is a detailed explanation of AI model lifecycle. Take a look…
i. Data Collection and Preparation
It involves extracting raw databases from various sources and the same data is then cleaned and restructured. Doing so allows businesses to transform the raw data into a high quality input database to train deep learning models.
Based on this, generative AI generates accurate and biased free results for businesses. Poor data preparation can result in objectionable and unfair outcomes, heightening the risk of deteriorating organisational performance.
ii. Training and Fine Tuning AI Models
It involves training an artificial intelligence model on a large dataset to learn patterns and structures. It is followed by a fine tuning process in which the model is trained on a smaller dataset for organizations. This way, developers ensure that the AI model understands how to generate outputs relevant to specific commands.
iii. Deployment and Continuous Monitoring
It refers to the process of using generative AI in the real environment to analyze its performance ensuring zero potential issues and high quality.
In case it shows any vulnerabilities, developers find underlying causes and biases to eliminate them so that the model can work with high productivity. As a result of this, organizations integrate safe and effective AI models into their existing workflows.
2. Ethical AI Management
Here is all about the ethical AI management. Take a look…
i. Ensuring Fairness and Accountability
Developers eliminate objectionable and biased approaches to AI models to foster transparency and accountability. They ensure the generative AI model meets ethical guidelines, preventing the risk of discriminatory results.
ii. Addressing Bias and Transparency in AI
To address bias and transparency in AI, developers prioritize high quality trained data that are prepared with organized data preparation. They constantly monitor potential biases, eliminating the threat of prejudiced outputs. Further they provide clear data sources to help team members understand their limitations and vulnerabilities.
3. Risk Management in Generative AI
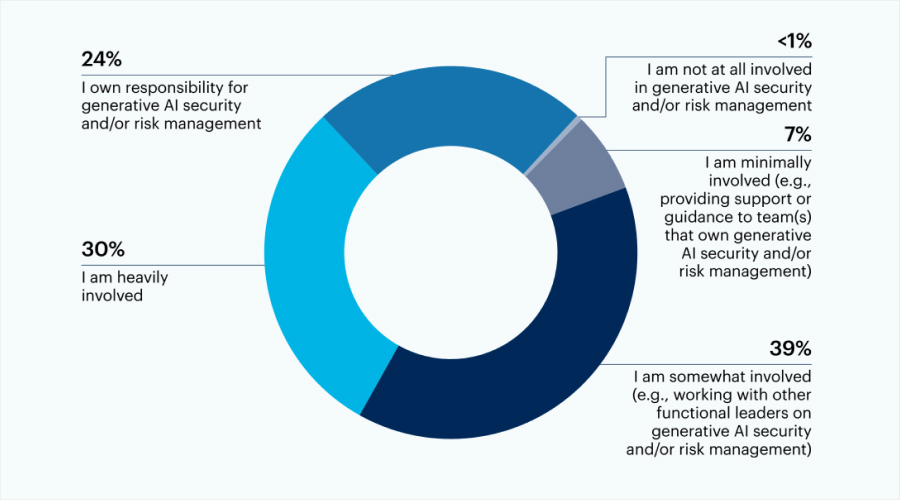
Let’s now take a look at the risk management in generative AI. Here we go…
i. Identifying AI Related Risks
To promote ethical and security concerns, developers address data privacy breaches, lack of transparency, adversarial attacks, corrupted data, etc. They ensure the trained dataset represents diverse populations, ensuring reduced biases and enhanced privacy.
ii. Mitigation Strategies for AI Failures
With a focus on robust data governance, bias mitigation techniques and clear AI governance frameworks, developers can eliminate potential risks, ensuring prejudiced free and safe outputs.
Let’s now move on to the key management strategies for business success. Here we go…
Generative AI Strategy for Business Success
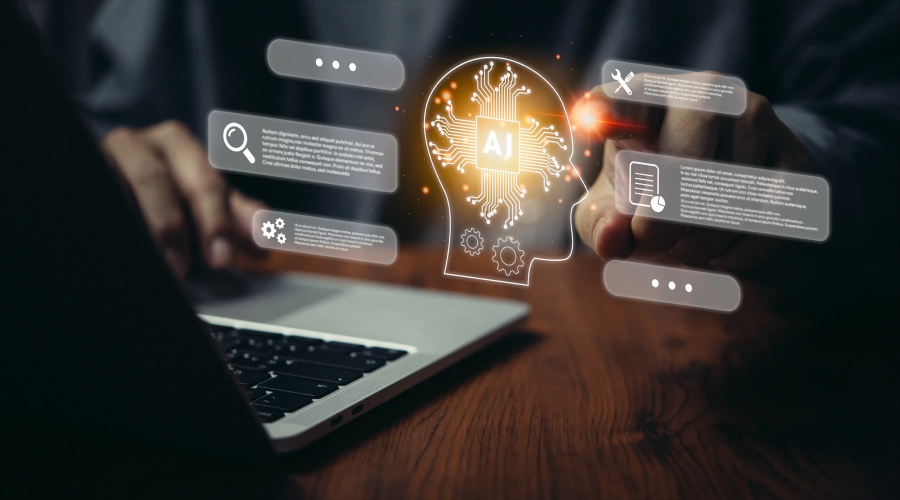
1. AI Resource Management
i. Infrastructure and Tools for AI
Tools like data management tools, data visualization tools and optimization and deployment tools play a crucial role in the infrastructure stage of artificial intelligence. These tools help manage, train, optimize and deploy data models to build a high performing generative AI model for progressive growth in businesses.
ii. Managing AI Talent and Expertise
Organizations should recruit candidates with high end technical skills to enhance AI productivity across diverse teams. Also, they should promote cross functional teams to promote greater employee engagement and help businesses reach business goals.
2. Product Management for Generative AI
i. AI Driven Products and Innovation
Generative AI automates repetitive tasks allowing team members to focus on building more innovative products. Not only does it accelerate business growth, but it also creates personalized and innovative customer focused solutions.
ii. Aligning AI Solutions with Business Needs
It involves careful selection of appropriate AI tools that can efficiently integrate into the existing workflows to help businesses achieve objectives. This way, AI solutions enhance productivity and improve customers’ experience.
Read on the next section to understand how integrating Generative AI into business operations can help businesses to operate seamlessly. Take a look…
Integrating Generative AI into Business Operations
1. AI in Marketing
Here is how AI can help businesses in marketing. Read on…
i. Personalizing Customer Interactions with AI
Generative AI model tailors their offerings to customers in real time, creating more relevant and engaging interactions. This way, it ensures the organization’s high productivity and customer satisfaction.
ii. Enhancing Marketing Campaigns with AI Technology
By automating content creation and leveraging insights into customer’s preferences, organizations build successful marketing campaigns to drive better results. This way, AI technology helps them achieve a competitive advantage.
2. Financial Management for AI Projects
AI comes with a price; hence, it is crucial to plan it strategically to make the most out of it. Here is how you can do it…
i. Budgeting for AI Initiatives
Investing in AI initiatives is a significant financial commitment as enterprises gain more than their invested money. However, there is no fixed price tag. Often, organizations invest depending on the scope of their plans, requisite technology for deployment and business objectives.
ii. Calculating AI ROI and Value
Calculating the ROI of artificial intelligence involves the cost of AI technology implementation, key performance indicators that show the influence of AI and net gain from the gained advantages. This way, it determines what financial value AI adds to organizations.
Overcoming Challenges in Generative AI Management
Integrating AI into your business operation can seem challenging at the outset. Here is how you can mitigate those challenges effortlessly. Take a look…
1. Technical Challenges in AI Deployment
Finding relevant and high quality labelled data is challenging for organizations when deploying AI for their work system. Poorly prepared data escalates the risk of data privacy and security vulnerabilities. It may deliver biased results. To get rid of the issue they need to source raw data from authentic and legal resources, preventing the risk of biased opinions.
As a result, they can prepare a safe and efficient database to train AI models to recognize accurate patterns and structures while generating results.
2. Ethical and Regulatory Concerns
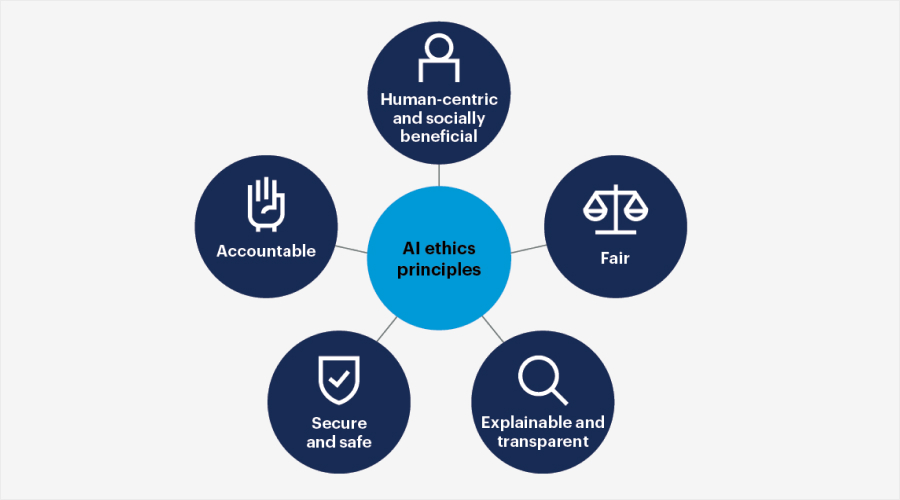
Often, unregulated generative AI models exacerbate issues like data privacy violations, copyright infringement, generating misleading information and lack of transparency. However, with a strong focus on build data protection program, anonymous data usage and compliance with AI regulations this situation can be reversed.
3. Organizational Change Management for AI Adoption
Embracing the transformative power of Generative AI is a game changer for the organization and team members. Often, they resist accepting these changes. To combat such issues the organization should focus on upskilling their employees and making them work with automation tools and software.
Also Read: AI in Business Examples
The Bottom Line
So, this is how you can use Generative AI mindfully. In a nutshell, adopting generative AI means automating repetitive tasks and business processes and exploring new opportunities to stay more productive and innovative in this competitive era. Generative AI can significantly influence businesses, ensuring unique offerings and improved efficiency.
However, businesses should understand its limitations, vulnerabilities, risk factors and potential biases to empower the workforce with a perfect AI model that can align with business goals.
Thanks for reading. Stay tuned for more such insightful articles!