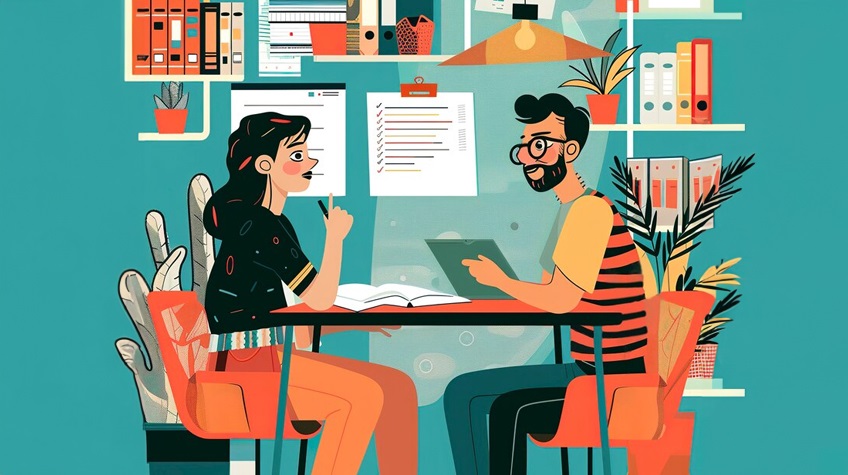
Hiring the right candidate for data annotation technology is very crucial to ensure precision and accuracy. However, finding the right candidate may seem like a task because this domain is relatively new, and very few candidates are available. Moreover, not all possess the knowledge and skillsets that are required for this data annotation technology. Also, you need to know what kind of interview questions and answers for data annotator you should ask to identify the right candidate for the role. So, whether you are looking for a data annotator for your company or are seeking a job in the data annotation field, this post is for you.
We have compiled a list of the top 25 data annotator interview questions and answers in this article to help you in your preparation. These questions will help you assess a candidate’s qualifications, skills, and strengths in the respective domain. So, let’s get straight to the interview questions and answers for the data annotator role. Here we go…
25 Interview Questions For The Data Annotator Role
Here is our rundown of the 25 most crucial questions, along with their answers for the data annotator profile, to help you choose the right candidate for your firm. Take a look…
Q1. Can you explain the importance of high-quality annotations to train machine algorithms?
High-quality annotations are very crucial as they are needed to train machine algorithms. They enhance their accuracy, speed, and AI predictive abilities.
Q2. How can you validate the machine algorithms?
Once machine algorithms are trained, they should go through the procedure of validation. This procedure ensures the efficiency of training against the set rules of data annotations. If the model is not valid, then, once again, it needs to undergo the process of training with high-quality annotated data.
Q3. How can data privacy be ensured while outsourcing data annotation?
Data privacy is a very heightened concern these days. Annotated data must be protected from malware attacks. It is the job of the data annotator to add more layers of security to the whole process. Anonymous internet use or masking of personally identifiable information (PII) is the best option.
Q4. Suggest alternatives to data annotation when it comes to validating predictive models.
Some of the alternatives for validating predictive models include Validation Application Block, Data Annotations Extensions Library, and Fluent Validation Library are some good alternatives.
Q5. Which metadata providers can you use with data annotation?
Three types of metadata providers can be used with data annotation:
- The .NET Framework type Description Provider
- The WCF Data Services Metadata Provider
- The .NET Framework Data Contract Serializer
Q6. Have you ever handled any difficult data annotation project? If yes, please explain.
Yes, I recently had the chance to work on the classification and image segmentation of medical imaging. It was quite a demanding task for me as it required meticulous attention to every detail of the images. However, I accomplished it with efficiency and accuracy. In addition, I discovered a fresh and creative method to enhance data annotation during this process.
Q7. Describe your experience in the domain of data annotation.
I have three years of experience in the domain of data annotation. During these three years, I have worked with industry experts on diverse data annotation projects. My specialization is in image classification and segmentation. However, I have done many successful projects on text annotation and audio annotation as well.
Q8. Do you know how to display currency values on a view page when employing data annotation?
Yes, I surely do! To display currency values on a view page while doing annotation, I should use the Display Format attribute. This is because this attribute allows you to specify the format that you want to choose for currency value.
Q9. What do you know about the job of a data annotator?
In the realm of artificial intelligence, data annotators play a crucial role. They should possess immense knowledge and expertise in order to label and tag data accurately. In addition, Data annotators must possess some key skills such as attention to minute details, innovative experiments, and effective communication. Having all these skill sets can help data annotators give the best outcomes and excel in the field. Furthermore, a good data annotator always keeps learning cutting-edge technology and stay updated with latest technology trends to be highly competitive and stay ahead.
Q10. How can you mitigate the risk of biased data annotation?
I strongly support work that is thoroughly researched and showcases innovative ideas. I dedicate countless hours to meticulously observing the intricate details of images or texts. I maintain a well-balanced approach for each data labeling and segmentation task. Throughout the entire procedure, my opinions remain completely separate from the data annotation process. By adopting this approach, I minimize the potential for data annotation to be influenced by bias.
Q11. How do you collaborate with your team to ensure the best machine algorithm outputs?
I know the importance of teamwork in Data annotation. I believe in exchanging ideas and sharing knowledge with my team members. I know that the success of any project depends on excellent teamwork. Furthermore, I actively participate in review sessions to ensure alignment and consistency across datasets.
Q12. What if your team members have discrepancies? How will you handle those discrepancies when you already have the pressure of delivering the project on time?
I believe in solving a problem through various perspectives. I strongly believe that every person has different perspective. Having differences in perspective can sometimes help find better solutions. In case of any discrepancy, I will take into account my team members’ approach also and try to understand things from their perspectives. However, if need be, I will discuss the issue with my senior team members as well.
Q13. Can you enable client-side processing while doing data annotation?
Yes, I can enable client-side processing while doing data annotation. By default, it remains on the server side. But, by enabling the client-side validation, I can make data annotation work with the client side.
Q14. How can client-side validation help you?
Client-side validation is beneficial in two ways. It reduces the server load and prevents the risk of malware-infected files in your system.
Q15. Is the conflict between ethical considerations and data annotation good?
No, there should not be any conflict between ethical considerations and data annotation. Ethical considerations are always non-negotiable in any organization. Growth or success should be aligned with ethical values.
Q16. What should you do to advance your learning in data annotation software or tools?
I am a good learner; I believe learning is a process. I actively participate in training sessions, attend webinars, and research the latest journals. By regularly attending industry forums, I can get updates on cutting-edge technology. Learning consistently can help me to stay abreast of the latest research and developments in this domain.
Q17. How can you accomplish the huge annotation of a complex dataset within the deadline?
I preferably do tasks according to the client’s priorities. To annotate a complex dataset, I employ the best strategies or software to accomplish this procedure without any flaw. Meeting deadlines does not compromise the quality. Furthermore, I believe in utilizing both, AI-automated tools and manual data annotation. I make use of AI-automated tool to automate repetitive tasks so as to save time and energy that I utilize in core activities to accomplish the project within the deadline.
Q18. Why is feedback necessary for team collaboration when it comes to data annotation?
Feedback widens the scope of research and improvements. With this, we can ensure new learnings and developments to make annotated data more effective and accurate.
Q19. Is low-quality data acceptable for data annotation?
No, low-quality data annotation is not at all acceptable as this can misguide machine algorithms. Misguided information can change the AI model’s predictive results. I should suggest changes to improve the quality of annotated data.
Q20. Why is data traceability essential throughout the data annotation process?
Data traceability ensures transparency, compliance, and adherence to the rules set against data annotation. A data annotator maintains it through meticulous labeling, audit controls, and version control.
Q21. What is data integrity in the data annotation process?
Data integrity is the accuracy, consistency, and reliability of annotated data. Maintaining data integrity ensures data safety throughout the outsourcing of data annotation projects.
Q22. What is the future of data annotation?
The future of data annotation technology is undoubtedly brighter! The surge for AI has led to an increased demand for data annotation tech in various industries. However, there are many annotated tools to annotate data. But, the most reliable and consistent data annotation is human-annotated data. So, the demand for highly qualified data annotators is going to go up for sure.
Q23. Why have you decided to become a data annotator?
I have decided to become a data annotator because I like to identify patterns and sequences in complex datasets. My interest in this domain made me learn diverse techniques of data annotation.
Q24. Are automated annotation tools reliable?
It is true that automated annotation tools accelerate productivity. However, their reliability is still questionable. Of course, we cannot completely rely on automated annotation tools. Several factors, such as the complexity of the dataset and the quality of the pre-existing data, can impact the performance of automated tools. Hence, we should use them at our discretion. The best way to use them in the process is to automate repetitive tasks. This will save a lot of time and energy that can be used in core tasks so that the project can be delivered on time and with precision.
Q25. How do you identify or rectify errors in annotated data?
Quality checks, cross-referencing annotations, and discrepancies can help identify errors in annotated data. Once errors are identified, reannotation can be done to rectify those errors.
Last Few Words
So, there you have it: the list of the top 25 data annotator’s interview questions and answers. Hopefully, this article has been informative for you and will help you ask the right questions to the candidates. The list covers a diverse range of topics, including technical skills, practical experience, and problem-solving abilities.
These questions can help you find the right candidate for the job. And if you are planning to appear for the interview, then it is also essential to familiarize yourself with these questions. Being aware of these questions, along with their answers, will allow you to confidently demonstrate your expertise in the field of data annotation. Know that preparing yourself prior to your interview is crucial to ensure you successfully crack the interview and get the job.